Data Mining and AI: Turning Data into Intelligence in the Digital Age
Data Mining represents a quiet revolution in the world of business and science, exploring vast amounts of data to uncover valuable patterns.
This article reveals how this process is carried out both efficiently and effectively.
We will explore the concept of Data Mining, understanding its stages, techniques, and its impact on the modern world.
What is Data Mining?
Data Mining is the process of discovering patterns and relationships in large data sets, acting like a detective analyzing data to uncover hidden mysteries.
It combines statistics, artificial intelligence (AI), and machine learning to identify hidden trends and patterns.
Beyond conventional data analysis, Data Mining automatically searches for anomalies and potential relationships, often revealing problems not identified by the user.
In summary, Data Mining leverages technology to unlock the hidden potential of data with minimal human intervention.
Data Mining finds practical applications in various fields, including marketing, healthcare, and business.
It predicts consumer behavior, identifies emerging patterns in various sectors, and discovers market opportunities.
By combining statistics, AI, and Machine Learning, Data Mining enables companies to enhance their competitiveness and maximize profits.
Data Mining Processes and Techniques
There are various approaches, but traditionally, speaking of Data Mining is about the most used methodology to perform it: Crisp-DM.
CRISP-DM (Cross-Industry Standard Process for Data Mining) is a widely-used model for guiding data mining projects, comprising six main stages, each with specific activities:
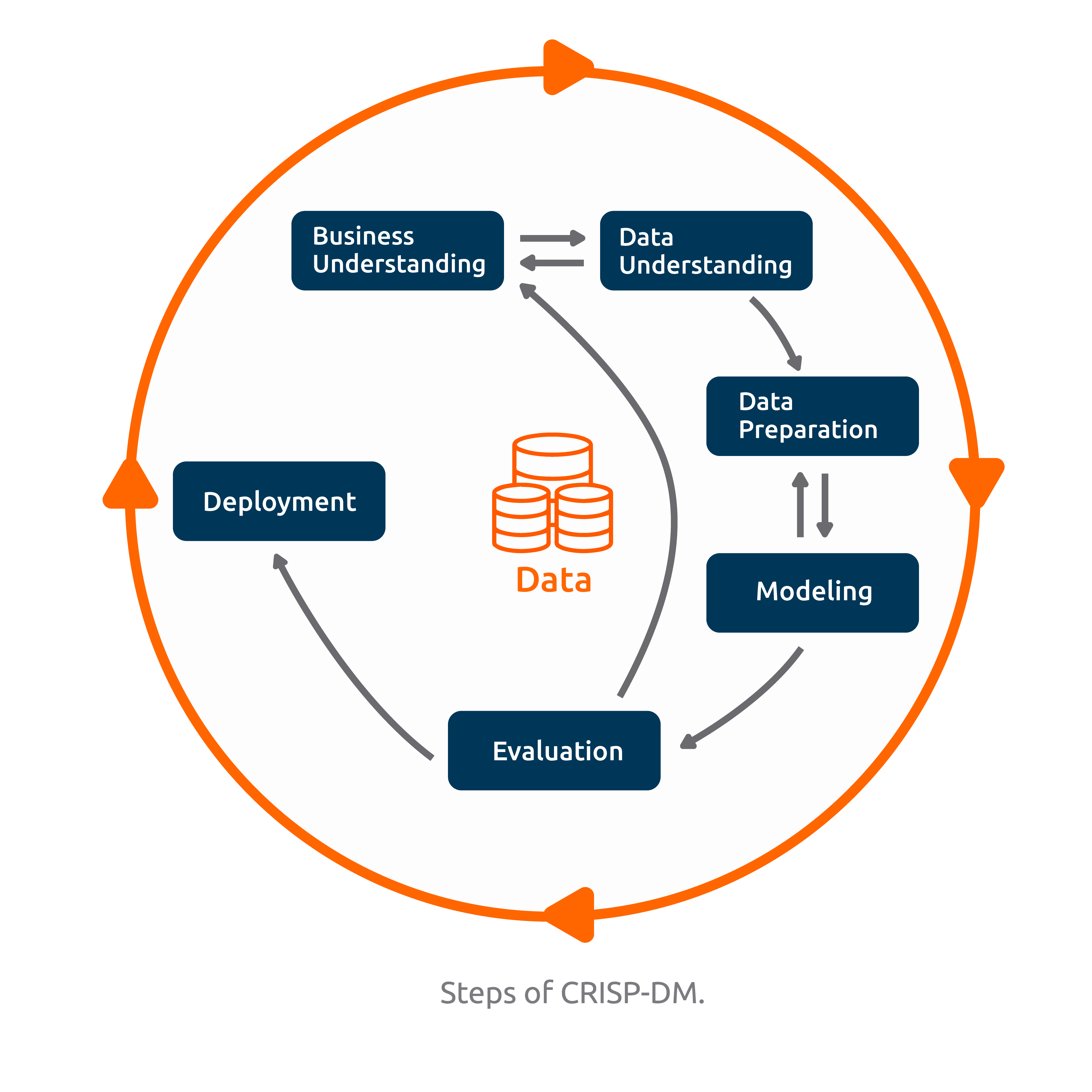
-
Business Understanding:
In this phase, it is fundamental to define the project’s objectives in business terms. This implies establishing clear goals and deeply understanding the needs of the stakeholders involved in the project.
To ensure that the project progresses in the right direction, it is essential to formulate a solid initial plan that allows achieving the previously defined objectives. This plan will serve as a guide for all subsequent stages of data mining, helping to keep the focus on the business goals set from the beginning.
-
Data Understanding:
It is essential to collect the relevant data for the project, gathering all the information necessary for the analysis.
This involves investigating the quality of the data, its content, and characteristics.
In addition, it is important to identify any potential problems that the data may present, such as missing values, noise (irrelevant or inaccurate information), or inconsistencies, allowing the project team to be aware of any limitations or challenges that may arise during the process.
-
Data Preparation:
The focus here is on preparing the data for analysis, ensuring it is in optimal form for effective use.
For this, it is necessary to:
Select and preprocess the relevant data for the analysis; Cleanse data, treat missing values, correct errors, and standardize formats; Transform the data to fit the specific needs of the mining model.
-
Modeling:
Here, the goal is to build and develop models that allow extracting useful information from the data through key steps:
Choose and apply modeling techniques appropriate to the data and project objectives. Train data mining models using selected algorithms. Evaluate and adjust the models to improve performance and accuracy.
Modeling is a critical step, as the resulting models are the basis for generating insights and making decisions.
Therefore, it is important to choose the right techniques, train the models appropriately, and ensure that they provide accurate and reliable results to meet the objectives of the data mining project.
-
Evaluation:
This involves a detailed analysis of the generated models to determine how effective they are in relation to the business objectives established at the beginning of the project.
The evaluation involves using appropriate metrics to measure the performance of the models.
Thus, it is possible to verify the results, whether they meet the criteria established in the business understanding phase.
-
Deployment:
The results obtained in the data analysis are put into practice in the business environment, creating tangible value and real impact.
This allows the integration of models or discoveries into business practice.
In this phase, strategies for monitoring are also developed to evaluate the performance of the models over time and ensure that they remain effective.
Technologies and Tools
Data Mining tools have evolved significantly, benefiting from the advancement of computers and sophisticated algorithms.
The integration of AI and Machine Learning has revolutionized the field, allowing deeper analyses and more accurate predictions.
Tools such as SQL, Python, Hadoop, and Apache Spark, as well as data capture, extraction, transformation, loading, and analysis services (such as Azure Data Factory, Azure ML Studio, AWS Glue, AWS Sagemaker, GCP Dataflow, GCP Vertex AI, among others), are essential, allowing complex and efficient analyses.
With the constant evolution of these technologies, the future of Data Mining is promising, opening new paths for discoveries and innovations.
Challenges and Ethics in Data Mining
Data Mining, although an extremely powerful tool, faces several challenges, especially regarding data quality and the accuracy of analytical models.
Data quality is fundamental, as inaccurate or poorly managed data can lead to incorrect conclusions or irrelevant insights.
Moreover, ensuring the accuracy of analytical models is crucial for the predictions and analyses to be reliable and useful in decision-making.
There is also a great concern regarding ethics in the use of Data Mining.
In a world where data is increasingly a valuable resource, issues related to data privacy and its responsible use become increasingly critical.
This includes concerns about how data is collected, stored, processed, and shared.
Companies and organizations must carefully navigate legal and regulatory issues, such as LGPD in Brazil and GDPR in the European Union, which establish strict guidelines for managing personal data.
The Future of Data Mining
Data Mining is leading the way to a more informed and efficient future.
With constantly evolving technologies, its possibilities continue to expand.
Generative Artificial Intelligence and Deep Learning enable new levels of data mining so that the concept of Data Mining takes more strength in the search for results from data.
It is not just a tool – it is a revolution in the interpretation and use of data.
With a deep understanding of how to extract value from data, Briteris offers customized solutions that seamlessly integrate Data Mining into your business.
Putting Data Mining into practice with Briteris means paving the way for more informed, efficient, and profitable decisions, positioning your company at the forefront of the digital age.